Plotting an ROC curve without context
How to do it...
An ROC curve is a diagnostic tool for any classifier without any context. No context means that we do not know yet which error type (FP or FN) is less desirable yet. Let us plot it right away using a vector of probabilities, y_pred_proba[:,1]:
from sklearn.metrics import roc_curve
fpr, tpr, ths = roc_curve(y_test, y_pred_proba[:,1])
plt.plot(fpr,tpr)
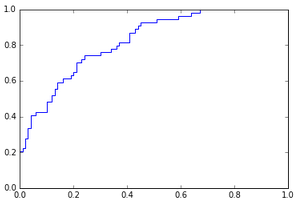
The ROC is a plot of the FPR (false alarms) in the x axis and TPR (finding everyone with the condition who really has it) in the y axis. Without context, it is a tool to measure classifier performance.