A traditional cookbook contains culinary recipes of interest to the authors, and helps readers expand their repertoire of foods to prepare. Many might believe that the end product of a recipe is the dish itself and one can read this book, in much the same way. Every chapter guides the reader through the application of the stages of the data science pipeline to different datasets with various goals. Also, just as in cooking, the final product can simply be the analysis applied to a particular set.
We hope that you will take a broader view, however. Data scientists learn by doing, ensuring that every iteration and hypothesis improves the practioner's knowledge base. By taking multiple datasets through the data science pipeline using two different programming languages (R and Python), we hope that you will start to abstract out the analysis patterns, see the bigger picture, and achieve a deeper understanding of this rather ambiguous field of data science.
We also want you to know that, unlike culinary recipes, data science recipes are ambiguous. When chefs begin a particular dish, they have a very clear picture in mind of what the finished product will look like. For data scientists, the situation is often different. One does not always know what the dataset in question will look like, and what might or might not be possible, given the amount of time and resources. Recipes are essentially a way to dig into the data and get started on the path towards asking the right questions to complete the best dish possible.
If you are from a statistical or mathematical background, the modeling techniques on display might not excite you per se. Pay attention to how many of the recipes overcome practical issues in the data science pipeline, such as loading large datasets and working with scalable tools to adapting known techniques to create data applications, interactive graphics, and web pages rather than reports and papers. We hope that these aspects will enhance your appreciation and understanding of data science and apply good data science to your domain.
Practicing data scientists require a great number and diversity of tools to get the job done. Data practitioners scrape, clean, visualize, model, and perform a million different tasks with a wide array of tools. If you ask most people working with data, you will learn that the foremost component in this toolset is the language used to perform the analysis and modeling of the data. Identifying the best programming language for a particular task is akin to asking which world religion is correct, just with slightly less bloodshed.
In this book, we split our attention between two highly regarded, yet very different, languages used for data analysis - R and Python and leave it up to you to make your own decision as to which language you prefer. We will help you by dropping hints along the way as to the suitability of each language for various tasks, and we'll compare and contrast similar analyses done on the same dataset with each language.
When you learn new concepts and techniques, there is always the question of depth versus breadth. Given a fixed amount of time and effort, should you work towards achieving moderate proficiency in both R and Python, or should you go all in on a single language? From our professional experiences, we strongly recommend that you aim to master one language and have awareness of the other. Does that mean skipping chapters on a particular language? Absolutely not! However, as you go through this book, pick one language and dig deeper, looking not only to develop conversational ability, but also fluency.
To prepare for this chapter, ensure that you have sufficient bandwidth to download up to several gigabytes of software in a reasonable amount of time.
Before we start installing any software, we need to understand the repeatable set of steps that we will use for data analysis throughout the book.
The following are the five key steps for data analysis:
- Acquisition: The first step in the pipeline is to acquire the data from a variety of sources, including relational databases, NoSQL and document stores, web scraping, and distributed databases such as HDFS on a Hadoop platform, RESTful APIs, flat files, and hopefully this is not the case, PDFs.
- Exploration and understanding: The second step is to come to an understanding of the data that you will use and how it was collected; this often requires significant exploration.
- Munging, wrangling, and manipulation: This step is often the single most time-consuming and important step in the pipeline. Data is almost never in the needed form for the desired analysis.
- Analysis and modeling: This is the fun part where the data scientist gets to explore the statistical relationships between the variables in the data and pulls out his or her bag of machine learning tricks to cluster, categorize, or classify the data and create predictive models to see into the future.
- Communicating and operationalizing: At the end of the pipeline, we need to give the data back in a compelling form and structure, sometimes to ourselves to inform the next iteration, and sometimes to a completely different audience. The data products produced can be a simple one-off report or a scalable web product that will be used interactively by millions.
Although the preceding list is a numbered list, don't assume that every project will strictly adhere to this exact linear sequence. In fact, agile data scientists know that this process is highly iterative. Often, data exploration informs how the data must be cleaned, which then enables more exploration and deeper understanding. Which of these steps comes first often depends on your initial familiarity with the data. If you work with the systems producing and capturing the data every day, the initial data exploration and understanding stage might be quite short, unless something is wrong with the production system. Conversely, if you are handed a dataset with no background details, the data exploration and understanding stage might require quite some time (and numerous non-programming steps, such as talking with the system developers).
The following diagram shows the data science pipeline:
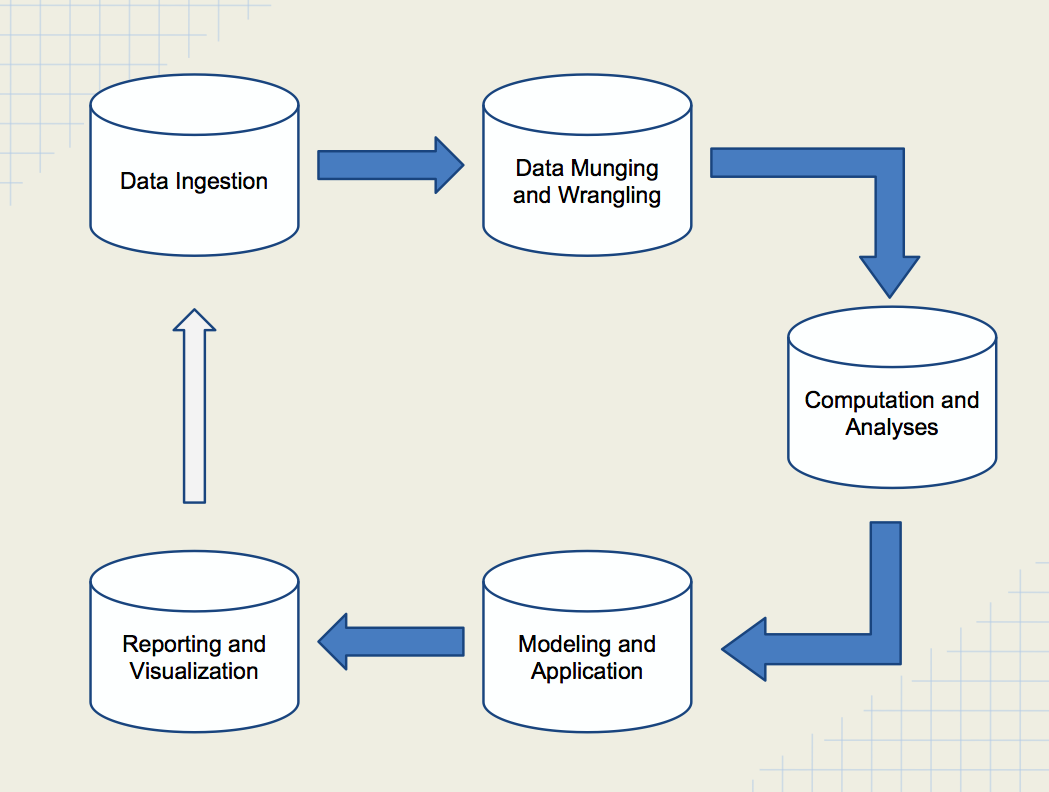
As you have probably heard or read by now, data munging or wrangling can often consume 80 percent or more of project time and resources. In a perfect world, we would always be given perfect data. Unfortunately, this is never the case, and the number of data problems that you will see is virtually infinite. Sometimes, a data dictionary might change or might be missing, so understanding the field values is simply not possible. Some data fields may contain garbage or values that have been switched with another field. An update to the web app that passed testing might cause a little bug that prevents data from being collected, causing a few hundred thousand rows to go missing. If it can go wrong, it probably did at some point; the data you analyze is the sum total of all of these mistakes.
The last step, communication and operationalization, is absolutely critical, but with intricacies that are not often fully appreciated. Note that the last step in the pipeline is not entitled data visualization and does not revolve around simply creating something pretty and/or compelling, which is a complex topic in itself. Instead, data visualizations will become a piece of a larger story that we will weave together from and with data. Some go even further and say that the end result is always an argument as there is no point in undertaking all of this effort unless you are trying to persuade someone or some group of a particular point.
Straight from the R project, R is a language and environment for statistical computing and graphics, and it has emerged as one of the de-facto languages for statistical and data analysis. For us, it will be the default tool that we use in the first half of the book.
Getting ready Make sure you have a good broadband connection to the Internet as you may have to download up to 200 MB of software.
Installing R is easy; use the following steps:
- Go to Comprehensive R Archive Network (CRAN) and download the latest release of R for your particular operating system:
- For Windows, go to http://cran.r-project.org/bin/windows/base/
- For Linux, go to http://cran.us.r-project.org/bin/linux/
- For Mac OS X, go to http://cran.us.r-project.org/bin/macosx/
As of June 2017, the latest release of R is Version 3.4.0 from April 2017.
- Once downloaded, follow the excellent instructions provided by CRAN to install the software on your respective platform. For both Windows and Mac, just double-click on the downloaded install packages.
- With R installed, go ahead and launch it. You should see a window similar to that shown in the following screenshot:
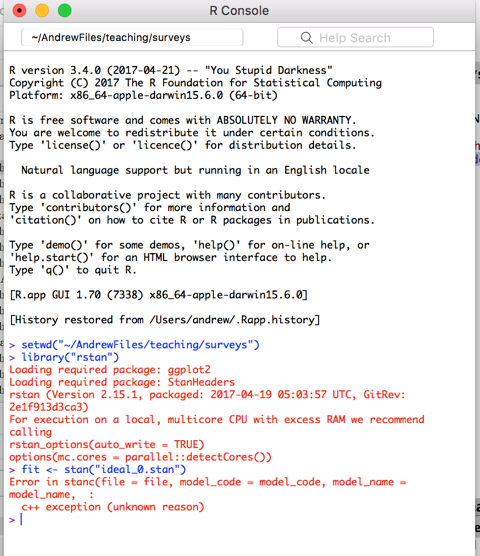
- An important modification of CRAN is available at https://mran.microsoft.com/ and it is a Microsoft contribution to R software. In fact, the authors are a fan of this variant and strongly recommend the Microsoft version as it has been demonstrated on multiple occasions that MRAN version is much faster than the CRAN version and all codes run the same on both the variants. So, there is a bonus reason to use MRAN R versions.
- You can stop at just downloading R, but you will miss out on the excellent Integrated Development Environment (IDE) built for R, called RStudio. Visit http://www.rstudio.com/ide/download/ to download RStudio, and follow the online installation instructions.
- Once installed, go ahead and run RStudio. The following screenshot shows one of our author's customized RStudio configurations with the
Console
panel in the upper-left corner, the editor in the upper-right corner, the current variable list in the lower-left corner, and the current directory in the lower-right corner:
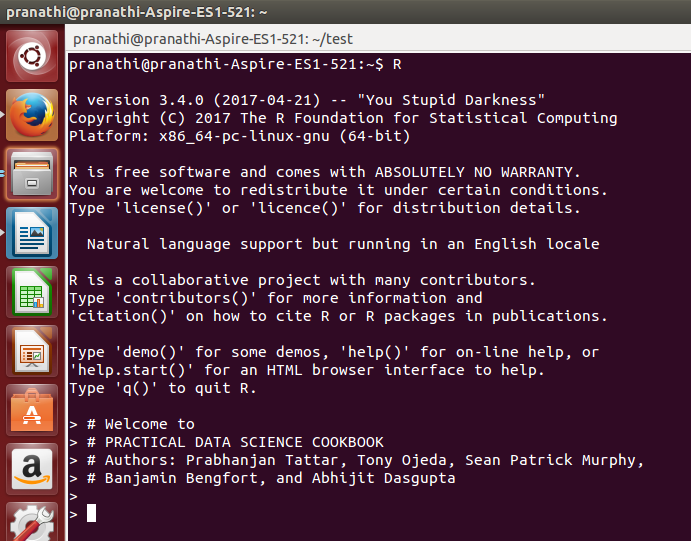
R is an interpreted language that appeared in 1993 and is an implementation of the S statistical programming language that emerged from Bell Labs in the '70s (S-PLUS is a commercial implementation of S). R, sometimes referred to as GNU S due to its open source license, is a domain-specific language (DSL) focused on statistical analysis and visualization. While you can do many things with R, not seemingly related directly to statistical analysis (including web scraping), it is still a domain-specific language and not intended for general-purpose usage.
R is also supported by CRAN, the Comprehensive R Archive Network ( http://cran.r-project.org/ ). CRAN contains an accessible archive of previous versions of R, allowing for analyses depending on older versions of the software to be reproduced. Further, CRAN contains hundreds of freely downloaded software packages, greatly extending the capability of R. In fact, R has become the default development platform for multiple academic fields, including statistics, resulting in the latest and greatest statistical algorithms being implemented first in R. The faster R versions are available in the Microsoft variants at https://mran.microsoft.com/.
RStudio ( http://www.rstudio.com/ ) is available under the GNU Affero General Public License v3 and is open source and free to use. RStudio, Inc., the company, offers additional tools and services for R as well as commercial support.
You can also refer to the following:
- Refer to the Getting Started with Rarticle at https://support.rstudio.com/hc/en-us/articles/201141096-Getting-Started-with-R
- Visit the home page for RStudio at http://www.rstudio.com/
- Refer to the Stages in the Evolution of S article at http://cm.bell-labs.com/cm/ms/departments/sia/S/history.html
- Refer to the A Brief History of S PS file at http://cm.bell-labs.com/stat/doc/94.11.ps
R has an incredible number of libraries that add to its capabilities. In fact, R has become the default language for many college and university statistics departments across the country. Thus, R is often the language that will get the first implementation of newly developed statistical algorithms and techniques. Luckily, installing additional libraries is easy, as you will see in the following sections.
R makes installing additional packages simple:
- Launch the R interactive environment or, preferably, RStudio.
- Let's install
ggplot2
. Type the following command, and then press the Enter key:
install.packages("ggplot2")
Note
Note that for the remainder of the book, it is assumed that, when we specify entering a line of text, it is implicitly followed by hitting the Return or Enter key on the keyboard
- You should now see text similar to the following as you scroll down the screen:
trying URL 'http://cran.rstudio.com/bin/macosx/contrib/3.0/ ggplot2_0.9.3.1.tgz'Content type 'application/x-gzip' length 2650041 bytes (2.5 Mb) opened URL ================================================== downloaded 2.5 Mb The downloaded binary packages are in /var/folders/db/z54jmrxn4y9bjtv8zn_1zlb00000gn/T//Rtmpw0N1dA/ downloaded_packages
- You might have noticed that you need to know the exact name, in this case,
ggplot2
, of the package you wish to install. Visit http://cran.us.r-project.org/web/packages/available_packages_by_name.html to make sure you have the correct name. - RStudio provides a simpler mechanism to install packages. Open up RStudio if you haven't already done so.
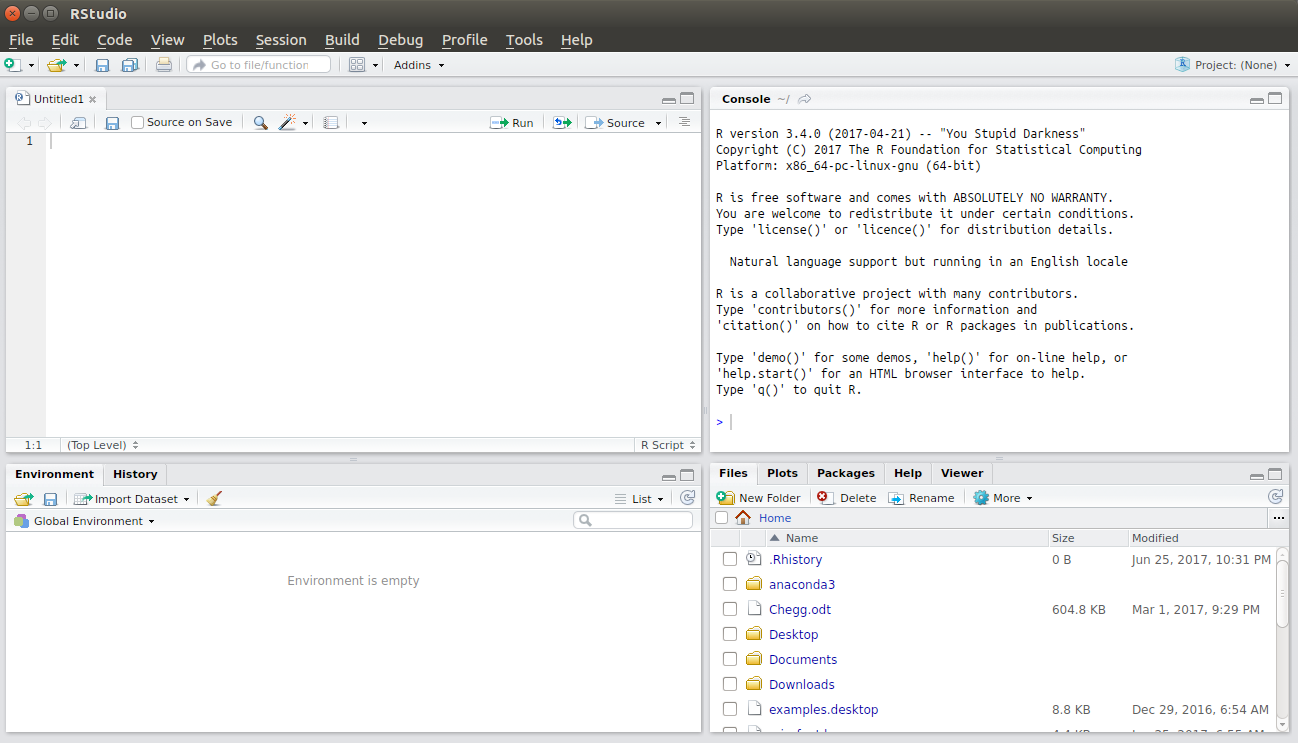
- Go to
Tools
in the menu bar and selectInstall Packages ...
. A new window will pop up, as shown in the following screenshot:
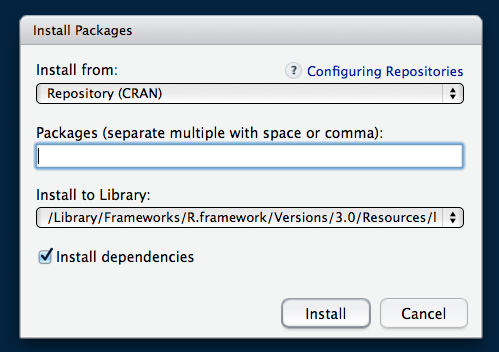
- As soon as you start typing in the
Packages
field, RStudio will show you a list of possible packages. The autocomplete feature of this field simplifies the installation of libraries. Better yet, if there is a similarly named library that is related, or an earlier or newer version of the library with the same first few letters of the name, you will see it. - Let's install a few more packages that we highly recommend. At the R prompt, type the following commands:
install.packages("lubridate")
install.packages("plyr")
install.packages("reshape2")
You can download the example code files for all Packt books you have purchased from your account at http://www.packtpub.com . If you purchased this book elsewhere, you can visit http://www.packtpub.com/support and register to have the files E-mailed directly to you.
Whether you use RStudio's graphical interface or the install.packages
command, you do the same thing. You tell R to search for the appropriate library built for your particular version of R. When you issue the command, R reports back the URL of the location where it has found a match for the library in CRAN and the location of the binary packages after download.
R's community is one of its strengths, and we would be remiss if we didn't briefly mention two things. R-bloggers is a website that aggregates R-related news and tutorials from over 750 different blogs. If you have a few questions on R, this is a great place to look for more information. The Stack Overflow site (
http://www.stackoverflow.com
) is a great place to ask questions and find answers on R using the tag rstats
.
Finally, as your prowess with R grows, you might consider building an R package that others can use. Giving an in-depth tutorial on the library building process is beyond the scope of this book, but keep in mind that community submissions form the heart of the R movement.
You can also refer to the following:
- Refer to the 10 R packages I wish I knew about earlier article at http://blog.yhathq.com/posts/10-R-packages-I-wish-I-knew-about-earlier.html
- Visit the R-bloggers website at http://www.r-bloggers.com/
- Refer to the Creating R Packages: A Tutorial at http://cran.r-project.org/doc/contrib/Leisch-CreatingPackages.pdf
- Refer to the Top 100 R packages for 2013 (Jan-May)! article at http://www.r-bloggers.com/top-100-r-packages-for-2013-jan-may/
- Visit the Learning R blog website at http://learnr.wordpress.com
Luckily for us, Python comes pre-installed on most versions of Mac OS X and many flavors of Linux (both the latest versions of Ubuntu and Fedora come with Python 2.7 or later versions out of the box). Thus, we really don't have a lot to do for this recipe, except check whether everything is installed.
For this book, we will work with Python 3.4.0.
Just make sure you have a good Internet connection in case we need to install anything.
Perform the following steps in the command prompt:
- Open a new Terminal window and type the following command:
which python
- If you have Python installed, you should see something like this:
/usr/bin/python
- Next, check which version you are running with the following command:
python --version
If you are planning on using OS X, you might want to set up a separate Python distribution on your machine for a few reasons. First, each time Apple upgrades your OS, it can and will obliterate your installed Python packages, forcing a reinstall of all previously installed packages. Secondly, new versions of Python will be released more frequently than Apple will update the Python distribution included with OS X. Thus, if you want to stay on the bleeding edge of Python releases, it is best to install your own distribution. Finally, Apple's Python release is slightly different from the official Python release and is located in a nonstandard location on the hard drive.
There are a number of tutorials available online to help walk you through the installation and setup of a separate Python distribution on your Mac. We recommend an excellent guide, available at http://docs.python-guide.org/en/latest/starting/install/osx/ , to install a separate Python distribution on your Mac.
You can also refer to the following:
- Refer to the Python For Beginners guide at http://www.python.org/about/gettingstarted/
- Refer to The Hitchhiker's Guide to Python at http://docs.python-guide.org/en/latest/
- Refer to the Python Development Environment onMac OS X Mavericks 10.9 article at http://hackercodex.com/guide/python-development-environment-on-mac-osx/
Installing Python on Windows systems is complicated, leaving you with three different options. First, you can choose to use the standard Windows release with executable installer from Python.org available at http://www.python.org/download/releases/ . The potential problem with this route is that the directory structure, and therefore, the paths for configuration and settings will be different from the standard Python installation. As a result, each Python package that was installed (and there will be many) might have path problems. Further, most tutorials and answers online won't apply to a Windows environment, and you will be left to your own devices to figure out problems. We have witnessed countless tutorial-ending problems for students who install Python on Windows in this way. Unless you are an expert, we recommend that you do not choose this option.
The second option is to install a prebundled Python distribution that contains all scientific, numeric, and data-related packages in a single install. There are two suitable bundles, one from Enthought and another from Continuum Analytics. Enthought offers the Canopy distribution of Python 3.5 in both 32- and 64-bit versions for Windows. The free version of the software, Canopy Express, comes with more than 50 Python packages pre-configured so that they work straight out of the box, including pandas, NumPy, SciPy, IPython, and matplotlib, which should be sufficient for the purposes of this book. Canopy Express also comes with its own IDE reminiscent of MATLAB or RStudio.
Continuum Analytics offers Anaconda, a completely free (even for commercial work) distribution of Python 2.7, and 3.6, which contains over 100 Python packages for science, math, engineering, and data analysis. Anaconda contains NumPy, SciPy, pandas, IPython, matplotlib, and much more, and it should be more than sufficient for the work that we will do in this book.
The third, and best option for purists, is to run a virtual Linux machine within Windows using the free VirtualBox (https://www.virtualbox.org/wiki/Downloads) from Oracle software. This will allow you to run Python in whatever version of Linux you prefer. The downside to this approach to that virtual machines tend to run a bit slower than native software, and you will have to get used to navigating via the Linux command line, a skill that any practicing data scientist should have.
Perform the following steps to install Python using VirtualBox:
- If you choose to run Python in a virtual Linux machine, visit https://www.virtualbox.org/wiki/Downloads to download VirtualBox from Oracle Software for free.
- Follow the detailed install instructions for Windows at https://www.virtualbox.org/manual/ch01.html#intro-installing.
- Continue with the instructions and walk through the sections entitled 1.6. Starting VirtualBox, 1.7 Creating your first virtual machine, and 1.8 Running your virtual machine.
- Once your virtual machine is running, head over to the Installing Python on Linux and Mac OS X recipe.
If you want to install Continuum Analytics' Anaconda distribution locally instead, follow these steps:
- If you choose to install Continuum Analytics' Anaconda distribution, go to http://continuum.io/downloads and select either the 64- or 32-bit version of the software (the 64-bit version is preferable) under Windows installers.
- Follow the detailed installation instructions for Windows at http://docs.continuum.io/anaconda/install.html.
For many readers, choosing between a prepackaged Python distribution and running a virtual machine might be easy based on their experience. If you are wrestling with this decision, keep reading. If you come from a windows-only background and/or don't have much experience with a *nix
command line, the virtual machine-based route will be challenging and will force you to expand your skill set greatly. This takes effort and a significant amount of tenacity, both useful for data science in general (trust us on this one). If you have the time and/or knowledge, running everything in a virtual machine will move you further down the path to becoming a data scientist and, most likely, make your code easier to deploy in production environments. If not, you can choose the backup plan and use the Anaconda distribution, as many people choose to do.
For the remainder of this book, we will always include Linux/Mac OS X-oriented Python package install instructions first and supplementary Anaconda install instructions second. Thus, for Windows users we will assume you have either gone the route of the Linux virtual machine or used the Anaconda distribution. If you choose to go down another path, we applaud your sense of adventure and wish you the best of luck! Let Google be with you.
You can also refer to the following:
- Refer to the Anaconda web page at https://store.continuum.io/cshop/anaconda/
- Visit the Enthought Canopy Express web page at https://www.enthought.com/canopy-express/
- Visit the VirtualBox website at https://www.virtualbox.org/
- Various installers of Python packages for Windows at http://www.lfd.uci.edu/~gohlke/pythonlibs
While Python is often said to have batteries included, there are a few key libraries that really take Python's ability to work with data to another level. In this recipe, we will install what is sometimes called the SciPy stack, which includes NumPy, SciPy, pandas, matplotlib, and Jupyter.
This recipe assumes that you have a standard Python installed.
Note
If, in the previous section, you decided to install the Anaconda distribution (or another distribution of Python with the needed libraries included), you can skip this recipe.
To check whether you have a particular Python package installed, start up your Python interpreter and try to import the package. If successful, the package is available on your machine. Also, you will probably need root access to your machine via the sudo
command.
The following steps will allow you to install the Python data stack on Linux:
- When installing this stack on Linux, you must know which distribution of Linux you are using. The flavor of Linux usually determines the package management system that you will be using, and the options include
apt-get
,yum
, andrpm
. - Open your browser and navigate to http://www.scipy.org/install.html , which contains detailed instructions for most platforms.
- These instructions may change and should supersede the instructions offered here, if different:
- Open up a shell.
- If you are using Ubuntu or Debian, type the following:
sudo apt-get install build-essential python-dev python- setuptools python-numpy python-scipy python-matplotlib ipython ipython-notebook python-pandas python-sympy python-nose
- If you are using Fedora, type the following:
sudo yum install numpy scipy python-matplotlib ipython python-pandas sympy python-nose
- You have several options to install the Python data stack on your Macintosh running OS X. These are:
- The first option is to download pre-built installers (
.dmg
) for each tool, and install them as you would any other Mac application (this is recommended). - The second option is if you have MacPorts, a command line-based system to install software, available on your system. You will also probably need XCode with the command-line tools already installed. If so, you can enter:
- The first option is to download pre-built installers (
sudo port install py27-numpy py27-scipy py27-matplotlib py27- ipython +notebook py27-pandas py27-sympy py27-nose
- As the third option, Chris Fonnesbeck provides a bundled way to install the stack on the Mac that is tested and covers all the packages we will use here. Refer to http://fonnesbeck.github.io/ScipySuperpack .
All the preceding options will take time as a large number of files will be installed on your system.
Installing the SciPy stack has been challenging historically due to compilation dependencies, including the need for Fortran. Thus, we don't recommend that you compile and install from source code, unless you feel comfortable doing such things.
Now, the better question is, what did you just install? We installed the latest versions of NumPy, SciPy, matplotlib, IPython, IPython Notebook, pandas, SymPy, and nose. The following are their descriptions:
- SciPy: This is a Python-based ecosystem of open source software for mathematics, science, and engineering and includes a number of useful libraries for machine learning, scientific computing, and modeling.
- NumPy: This is the foundational Python package providing numerical computation in Python, which is C-like and incredibly fast, particularly when using multidimensional arrays and linear algebra operations. NumPy is the reason that Python can do efficient, large-scale numerical computation that other interpreted or scripting languages cannot do.
- matplotlib: This is a well-established and extensive 2D plotting library for Python that will be familiar to MATLAB users.
- IPython: This offers a rich and powerful interactive shell for Python. It is a replacement for the standard Python Read-Eval-Print Loop (REPL), among many other tools.
- Jupyter Notebook: This offers a browser-based tool to perform and record work done in Python with support for code, formatted text, markdown, graphs, images, sounds, movies, and mathematical expressions.
- pandas: This provides a robust data frame object and many additional tools to make traditional data and statistical analysis fast and easy.
- nose: This is a test harness that extends the unit testing framework in the Python standard library.
We will discuss the various packages in greater detail in the chapter in which they are introduced. However, we would be remiss if we did not at least mention the Python IDEs. In general, we recommend using your favorite programming text editor in place of a full-blown Python IDE. This can include the open source Atom from GitHub, the excellent Sublime Text editor, or TextMate, a favorite of the Ruby crowd. Vim and Emacs are both excellent choices not only because of their incredible power but also because they can easily be used to edit files on a remote server, a common task for the data scientist. Each of these editors is highly configurable with plugins that can handle code completion, highlighting, linting, and more. If you must have an IDE, take a look at PyCharm (the community edition is free) from the IDE wizards at JetBrains, Spyder, and Ninja-IDE. You will find that most Python IDEs are better suited for web development as opposed to data work.
You can also take a look at the following for reference:
- For more information on pandas, refer to the Python Data Analysis Library article at http://pandas.pydata.org/
- Visit the NumPy website at http://www.numpy.org/
- Visit the SciPy website at http://www.scipy.org/
- Visit the matplotlib website at http://matplotlib.org/
- Visit the IPython website at http://ipython.org/
- Refer the History of SciPy article at http://wiki.scipy.org/History_of_SciPy
- Visit the MacPorts home page at http://www.macports.org/
- Visit the XCode web page at https://developer.apple.com/xcode/features/
- Visit the XCode download page at https://developer.apple.com/xcode/downloads/
There are a few additional Python libraries that you will need throughout this book. Just as R provides a central repository for community-built packages, so does Python in the form of the Python Package Index (PyPI). As of August 28, 2014, there were 48,054 packages in PyPI.
A reasonable Internet connection is all that is needed for this recipe. Unless otherwise specified, these directions assume that you are using the default Python distribution that came with your system, and not Anaconda.
The following steps will show you how to download a Python package and install it from the command line:
- Download the source code for the package in the place you like to keep your downloads.
- Unzip the package.
- Open a terminal window.
- Navigate to the base directory of the source code.
- Type in the following command:
python setup.py install
- If you need root access, type in the following command:
sudo python setup.py install
To use pip, the contemporary and easiest way to install Python packages, follow these steps:
- First, let's check whether you have pip already installed by opening a terminal and launching the Python interpreter. At the interpreter, type:
>>>import pip
- If you don't get an error, you have pip installed and can move on to step 5. If you see an error, let's quickly install pip.
- Download the
get-pip.py
file from https://raw.github.com/pypa/pip/master/contrib/get-pip.py onto your machine. - Open a terminal window, navigate to the downloaded file, and type:
python get-pip.py
Alternatively, you can type in the following command:
sudo python get-pip.py
- Once pip is installed, make sure you are at the system command prompt.
- If you are using the default system distribution of Python, type in the following:
pip install networkx
Alternatively, you can type in the following command:
sudo pip install networkx
- If you are using the Anaconda distribution, type in the following command:
conda install networkx
- Now, let's try to install another package,
ggplot
. Regardless of your distribution, type in the following command:
pip install ggplot
Alternatively, you can type in the following command:
sudo pip install ggplot
You have at least two options to install Python packages. In the preceding old fashioned way, you download the source code and unpack it on your local computer. Next, you run the included setup.py
script with the install
flag. If you want, you can open the setup.py
script in a text editor and take a more detailed look at exactly what the script is doing. You might need the sudo
command, depending on the current user's system privileges.
As the second option, we leverage the pip installer, which automatically grabs the package from the remote repository and installs it to your local machine for use by the system-level Python installation. This is the preferred method, when available.
The pip
is capable, so we suggest taking a look at the user guide online. Pay special attention to the very useful pip freeze > requirements.txt
functionality so that you can communicate about external dependencies with your colleagues.
Finally, conda
is the package manager and pip replacement for the Anaconda Python distribution or, in the words of its home page, a cross-platform, Python-agnostic binary package manager. Conda has some very lofty aspirations that transcend the Python language. If you are using Anaconda, we encourage you to read further on what conda
can do and use it, and not pip, as your default package manager.
You can also refer to the following:
- Refer to the pip User Guide at http://www.pip-installer.org/en/latest/user_guide.html
- Visit the Conda home page at http://conda.pydata.org
- Refer to the Conda blog posts from Continuum Blog at http://www.continuum.io/blog/conda
virtualenv is a transformative Python tool. Once you start using it, you will never look back. virtualenv creates a local environment with its own Python distribution installed. Once this environment is activated from the shell, you can easily install packages using pip install
into the new local Python.
At first, this might sound strange. Why would anyone want to do this? Not only does this help you handle the issue of package dependencies and versions in Python but also allows you to experiment rapidly without breaking anything important. Imagine that you build a web application that requires Version 0.8 of the awesome_template
library, but then your new data product needs the awesome_template
library Version 1.2. What do you do? With virtualenv, you can have both.
As another use case, what happens if you don't have admin privileges on a particular machine? You can't install the packages using sudo pip install
required for your analysis so what do you do? If you use virtualenv, it doesn't matter.
Virtual environments are development tools that software developers use to collaborate effectively. Environments ensure that the software runs on different computers (for example, from production to development servers) with varying dependencies. The environment also alerts other developers to the needs of the software under development. Python's virtualenv ensures that the software created is in its own holistic environment, can be tested independently, and built collaboratively.
Install and test the virtual environment using the following steps:
- Open a command-line shell and type in the following command:
pip install virtualenv
Alternatively, you can type in the following command:
sudo pip install virtualenv
- Once installed, type
virtualenv
in the command window, and you should be greeted with the information shown in the following screenshot:
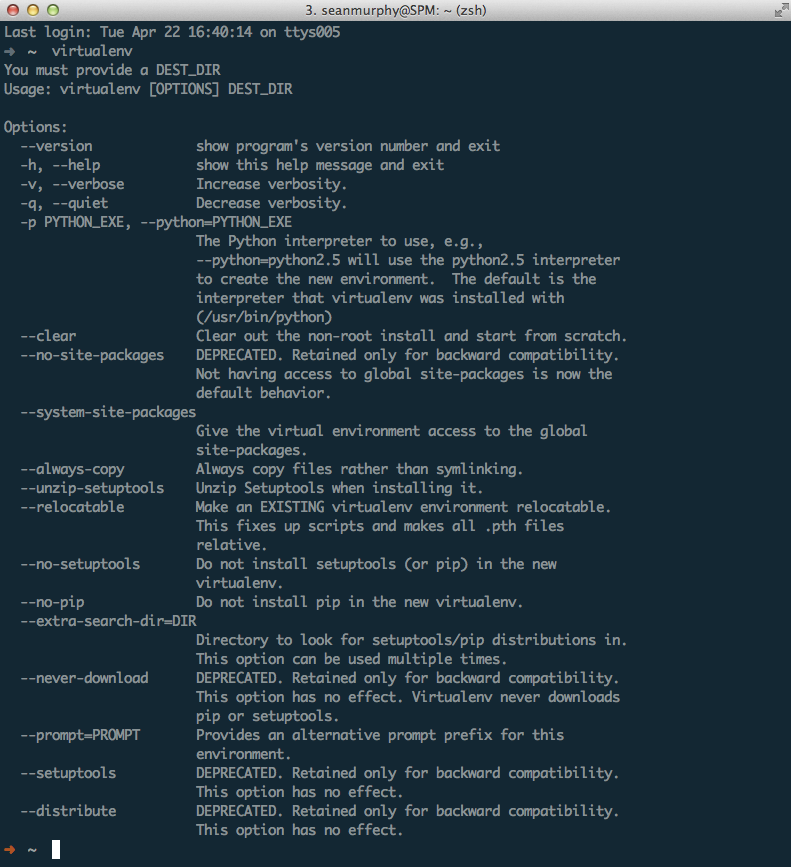
- Create a temporary directory and change location to this directory using the following commands:
mkdir temp
cd temp
- From within the directory, create the first virtual environment named
venv
:
virtualenv venv
- You should see text similar to the following:
New python executable in venv/bin/python
Installing setuptools, pip...done.
- The new local Python distribution is now available. To use it, we need to activate
venv
using the following command:
source ./venv/bin/activate
- The activated script is not executable and must be activated using the
source
command. Also, note that your shell's command prompt has probably changed and is prefixed withvenv
to indicate that you are now working in your new virtual environment. - To check this fact, use
which
to see the location of Python, as follows:
which python
You should see the following output:
/path/to/your/temp/venv/bin/python
So, when you type python
once your virtual environment is activated, you will run the local Python.
- Next, install something by typing the following:
pip install flask
Flask is a micro-web framework written in Python; the preceding command will install a number of packages that Flask uses.
- Finally, we demonstrate the versioning power that virtual environment and pip offer, as follows:
pip freeze > requirements.txt
cat requirements.txt
This should produce the following output:
Flask==0.10.1
Jinja2==2.7.2
MarkupSafe==0.19
Werkzeug==0.9.4
itsdangerous==0.23
wsgiref==0.1.2
- Note that not only the name of each package is captured, but also the exact version number. The beauty of this
requirements.txt
file is that, if we have a new virtual environment, we can simply issue the following command to install each of the specified versions of the listed Python packages:
pip install -r requirements.txt
- To deactivate your virtual environment, simply type the following at the shell prompt:
deactivate
virtualenv creates its own virtual environment with its own installation directories that operate independently from the default system environment. This allows you to try out new libraries without polluting your system-level Python distribution. Further, if you have an application that just works and want to leave it alone, you can do so by making sure the application has its own virtualenv
.
virtualenv
is a fantastic tool, one that will prove invaluable to any Python programmer. However, we wish to offer a note of caution. Python provides many tools that connect to C-shared objects in order to improve performance. Therefore, installing certain Python packages
, such as NumPy and SciPy, into your virtual environment may require external dependencies to be compiled and installed, which are system specific. Even when successful, these compilations can be tedious, which is one of the reasons for maintaining a virtual environment. Worse, missing dependencies will cause compilations to fail, producing errors that require you to troubleshoot alien error messages, dated make files, and complex dependency chains. This can be daunting even to the most veteran data scientist.
A quick solution is to use a package manager to install complex libraries into the system environment (aptitude or Yum for Linux, Homebrew or MacPorts for OS X, and Windows will generally already have compiled installers). These tools use precompiled forms of the third-party packages. Once you have these Python packages installed in your system environment, you can use the --system-site-packages
flag when initializing a virtualenv
. This flag tells the virtualenv
tool to use the system site packages already installed and circumvents the need for an additional installation that will require compilation. In order to nominate packages particular to your environment that might already be in the system (for example, when you wish to use a newer version of a package), use pip install -I
to install dependencies into virtualenv
and ignore the global packages. This technique works best when you only install large-scale packages on your system, but use virtualenv
for other types of development.
For the rest of the book, we will assume that you are using a virtualenv
and have the tools mentioned in this chapter ready to go. Therefore, we won't enforce or discuss the use of virtual environments in much detail. Just consider the virtual environment as a safety net
that will allow you to perform the recipes listed in this book in isolation.
You can also refer to the following:
- Read an introduction to virtualenv at http://www.virtualenv.org/en/latest/virtualenv.html
- Explore virtualenvwrapper at http://virtualenvwrapper.readthedocs.org/en/latest/
- Explore virtualenv at https://pypi.python.org/pypi/virtualenv